Unleashing the Transformative Power of AI in Networking: A Paradigm Shift
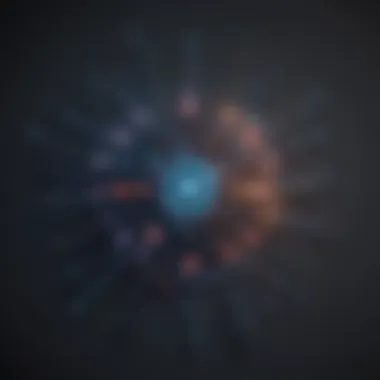
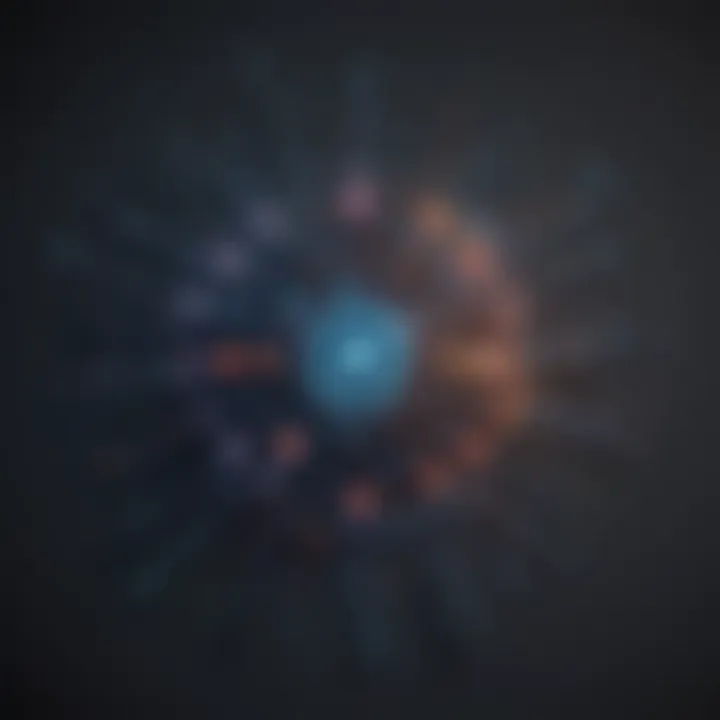
Overview of Cyber Security Threats
Artificial intelligence in networking is a field with immense potential; however, it also presents unique cybersecurity challenges. Various cyber threats, such as malware, phishing, and ransomware, pose significant risks to network security. With AI-driven advancements, these threats evolve in complexity, making them harder to detect and mitigate. Furthermore, statistics on cyber attacks reveal a growing trend of sophisticated cybercriminal activities targeting networks globally. Real-life examples of security breaches underscore the urgent need for robust cybersecurity measures to safeguard organizations from crippling data breaches and financial losses.
Best Practices for Online Security
In the realm of AI-powered networking, adhering to best practices for online security is paramount to mitigate risks and enhance resilience against cyber threats. Strong password creation and management techniques play a critical role in safeguarding network resources from unauthorized access. Regularly implementing software updates and patches is essential to address vulnerabilities and strengthen network defenses. The implementation of two-factor authentication adds an extra layer of security, making it harder for malicious actors to compromise network access.
Reviews of Security Tools
As organizations navigate the dynamic landscape of AI-integrated networking, evaluating the effectiveness of security tools becomes crucial. Antivirus software plays a pivotal role in detecting and eliminating malware, offering essential protection against cyber threats. Comparing firewall protection mechanisms helps organizations choose robust solutions that fortify network boundaries and prevent unauthorized access. Assessing the utility of password managers streamlines secure password storage and access, enhancing overall password security and usability.
Tips for Ensuring Online Privacy
Maintaining online privacy in the age of AI-enhanced networking requires vigilant efforts to safeguard personal data and sensitive information. Leveraging Virtual Private Networks (VPNs) ensures secure browsing by encrypting network traffic and shielding user identities from online surveillance. Adhering to privacy settings on social media platforms limits data exposure and minimizes potential privacy risks associated with online interactions. Employing encryption protocols and ensuring data encryption during online transactions bolster privacy protection and mitigate risks of data interception.
Educational Resources and Guides
Empowering individuals with education and guidance on online security practices is integral to fostering a culture of cyber resilience. Access to instructional resources, such as how-to articles on setting up encryption tools, equips users with practical knowledge to enhance data security measures. Step-by-step guides on recognizing and thwarting phishing emails aid in building user awareness and resilience against social engineering attacks. Cheat sheets encapsulating quick tips and strategies for enhancing online security serve as handy references for individuals seeking to fortify their digital defenses.
Introduction to Artificial Intelligence in Networking
Artificial Intelligence (AI) is revolutionizing the field of networking, ushering in a new era of efficiency and innovation. In this article, we delve into the transformative impact of AI on network operations, exploring how this technology is reshaping the way organizations optimize their networks, bolster cybersecurity measures, and handle vast amounts of data. AI is not just a buzzword but a fundamental game-changer in the networking landscape. By infusing intelligence into network management systems, AI enables organizations to streamline operations, predict maintenance needs, and ensure seamless traffic flow. This increased automation and predictive capacity are invaluable in managing the complexities of modern networks swiftly and effectively. The use of AI in dynamic bandwidth allocation further enhances network performance by allowing for real-time resource distribution based on traffic demands and prioritizing the quality of service. Enterprises are now leveraging AI to stay ahead of the competition and meet the evolving demands of a digital world, where connectivity and speed are paramount.
Understanding the Role of AI in Network Optimization
Artificial Intelligence (AI) plays a pivotal role in optimizing networks, offering a wealth of benefits and considerations that are paramount to the efficacy and success of modern networking systems. By harnessing AI technologies, organizations can achieve unprecedented levels of efficiency, security, and innovation in managing their networks. The strategic integration of AI in network optimization enables proactive problem-solving, intelligent decision-making, and streamlined operations.
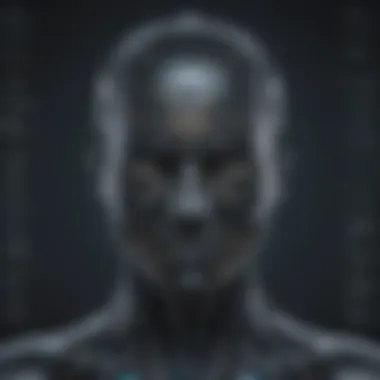
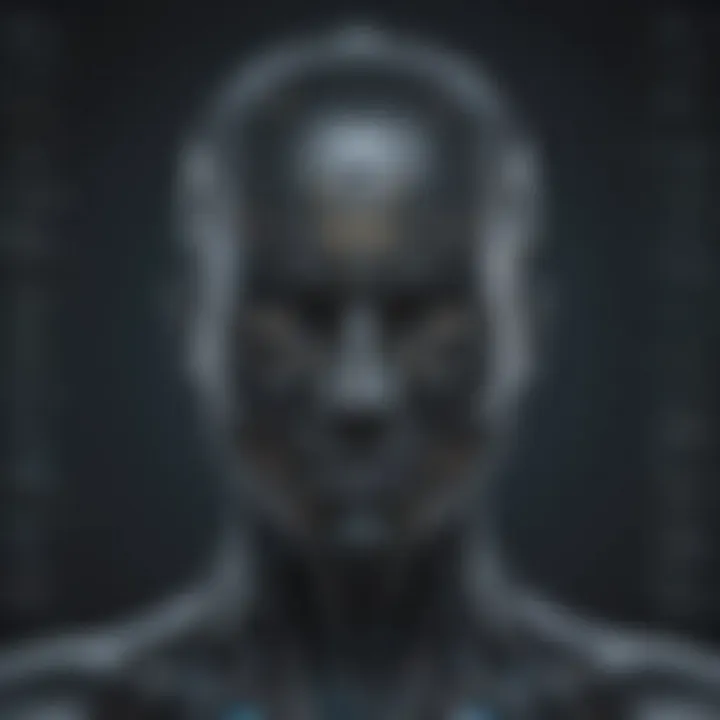
AI-Powered Network Management Systems
In the realm of network optimization, AI-powered network management systems stand out as game-changers, revolutionizing how traffic flow is optimized and maintenance strategies are predicted. The optimization of traffic flow involves the real-time analysis of network data to ensure smooth and efficient data transmission. This process enhances network performance, reduces latency, and prioritizes critical data delivery. Despite its advantages, optimizing traffic flow may face challenges related to complex network structures and high data volumes.
Predictive maintenance strategies leverage AI algorithms to forecast potential network disruptions and equipment failures. By identifying patterns and anomalies in network behavior, predictive maintenance minimizes downtime, enhances system reliability, and prolongs hardware lifespan effectively. However, these strategies require continuous refinement and monitoring to align with evolving network dynamics.
Dynamic Bandwidth Allocation
Dynamic bandwidth allocation within AI-driven networks entails real-time resource allocation and quality of service enhancements. Real-time resource allocation optimizes network resources based on demand fluctuations, ensuring optimal utilization without over-provisioning. This approach enhances scalability, flexibility, and cost-efficiency in network operations. Nevertheless, challenges may arise in balancing resource allocation dynamically across diverse network components.
Quality of service enhancements prioritize delivering consistent and reliable network services to users. By allocating resources based on service requirements, organizations can ensure high performance, low latency, and minimal packet loss. While quality of service enhancements elevate user experience, sustaining consistent service levels demands sophisticated network monitoring and adaptive resource allocation mechanisms.
Enhancing Cybersecurity Through AI
Integrating Artificial Intelligence (AI) into cybersecurity measures is a pivotal focus in modern networking paradigms. The symbiotic relationship between AI and cybersecurity enhances the overall resilience of networks against evolving threats and vulnerabilities. By leveraging AI algorithms and machine learning capabilities, organizations can proactively detect and mitigate potential security incidents, fortifying their defense mechanisms and reducing the risk of data breaches and cyber attacks.
In this article, the discussion around Enhancing Cybersecurity Through AI highlights the crucial role that AI plays in bolstering network security through advanced threat detection and automated incident response mechanisms. By embracing AI-driven cybersecurity solutions, organizations can achieve real-time threat mitigation and security orchestration to safeguard sensitive data and preserve the integrity of their network infrastructure. The meticulous integration of AI technologies empowers network administrators with the tools and insights needed to combat sophisticated cyber threats effectively.
AI-Driven Threat Detection
AI-powered threat detection mechanisms such as Behavioral Analysis and Anomaly Detection serve as the frontline defense against malicious activities within network environments. Behavioral Analysis scrutinizes user behavior patterns and network activities to identify deviations from normal actions, enabling early detection of insider threats or suspicious activities. Its nuanced approach to anomaly identification enhances the accuracy of threat detection by pinpointing irregularities that evade traditional security measures.
On the other hand, Anomaly Detection focuses on detecting deviations from expected behaviors or network traffic patterns, signaling potential security risks or cyber threats. Its ability to differentiate between normal and anomalous activities allows organizations to proactively address security incidents before they escalate, mitigating potential damages and minimizing disruption to network operations. Despite its effectiveness in early threat detection, Anomaly Detection may generate false positives, necessitating ongoing refinement and calibration for optimal performance.
Automated Incident Response
The realm of automated incident response in cybersecurity encompasses Security Orchestration and Incident Mitigation Strategies as key components in bolstering defensive capabilities. Security Orchestration streamlines incident response processes by automating repetitive tasks, facilitating rapid threat containment, and coordination across security tools. This orchestrated approach enhances the efficiency of incident handling and enables timely responses to mitigate security breaches effectively.
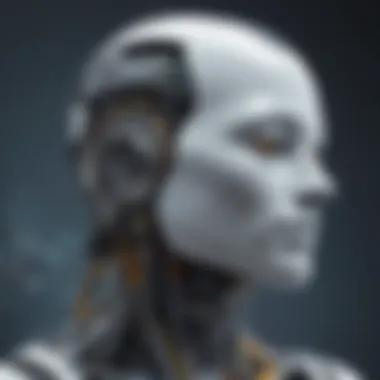
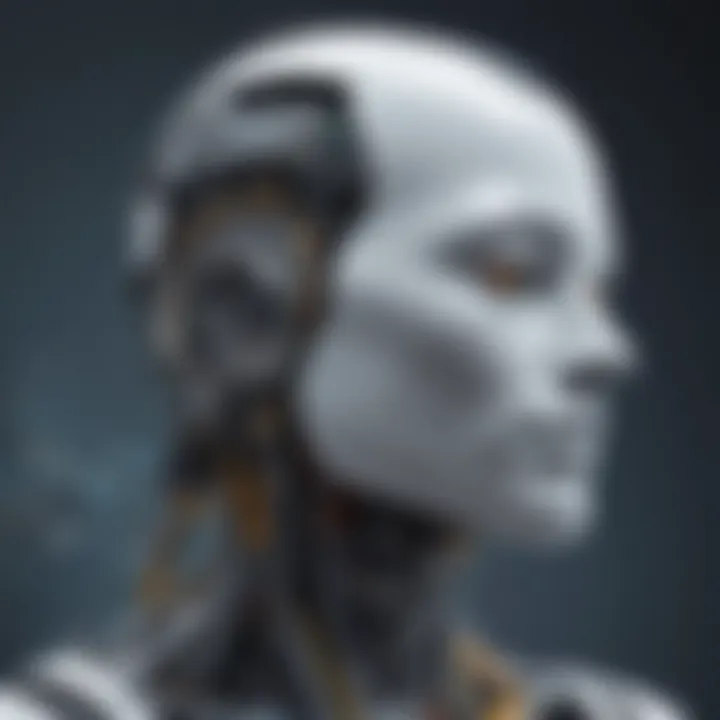
On the other hand, Incident Mitigation Strategies focus on preemptive measures and targeted actions to mitigate the impact of security incidents post-detection. By implementing proactive mitigation strategies, organizations can reduce the vulnerabilities exposed during an attack, limiting potential damages and restoring network functionality efficiently. The strategic deployment of incident mitigation tactics relies on data-driven insights and AI-driven analytics to optimize response efforts and enhance overall incident response readiness.
The Evolution of Artificial Intelligence (AI) in Data Management
Artificial intelligence (AI) is revolutionizing data management practices, playing a crucial role in modern networking. In this article, delving deep into the topic of AI in data management is imperative to grasp the transformative impact it has on network operations. AI brings efficiency, security, and innovation to data handling processes.
Predictive Analytics in Network Data Handling
Capacity Planning:
Capacity planning is a vital aspect of predictive analytics in network data handling. It involves forecasting future capacity requirements based on historical data and trends. The key characteristic of capacity planning is its ability to preemptively address potential bottlenecks or overloads within the network infrastructure. This proactive approach aids in ensuring smooth network operations, optimizing resource utilization effectively. Despite its benefits, capacity planning requires accurate data and foresight to be truly advantageous in optimizing network performance.
Performance Optimization:
Performance optimization is another crucial component of predictive analytics in data handling. It focuses on enhancing the overall efficiency and speed of data processing within the network. The primary characteristic of performance optimization is its ability to streamline operations and minimize latency for better user experience. By fine-tuning network parameters and algorithms, performance optimization contributes significantly to maximizing the network's capabilities. However, the challenge lies in balancing optimization efforts with potential complexities that may arise, requiring continuous adjustments and monitoring for optimal results.
AI-Driven Data Security Protocols
Encryption Techniques:
Encryption techniques form a fundamental part of AI-driven data security protocols, safeguarding sensitive information from unauthorized access or manipulation. The key characteristic of encryption techniques is their ability to convert data into a secure format that can only be decoded with the appropriate key. This method ensures confidentiality and integrity in data transmission and storage processes. One unique feature of encryption techniques is end-to-end encryption, which protects data throughout its entire journey across the network. However, implementing encryption may introduce processing overhead and complexity, impacting performance in high-throughput environments.
Access Control Mechanisms:
Access control mechanisms play a pivotal role in regulating and managing user permissions within network infrastructures. The key characteristic of access control mechanisms lies in their ability to dictate who can access specific resources or data within the network environment. By implementing granular access controls, organizations can enforce security policies and prevent unauthorized activities. One unique feature of access control mechanisms is role-based access control, which assigns privileges based on predefined roles or responsibilities. However, overly restrictive access controls can hinder operational efficiency, necessitating a fine balance between security and usability in network settings.
Future Perspectives and Trends in AI Networking
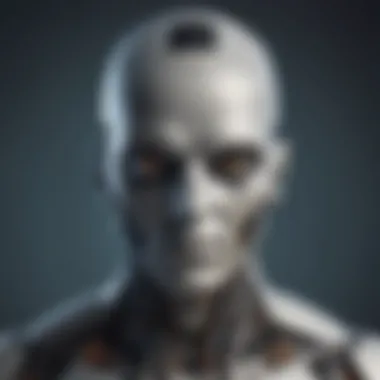
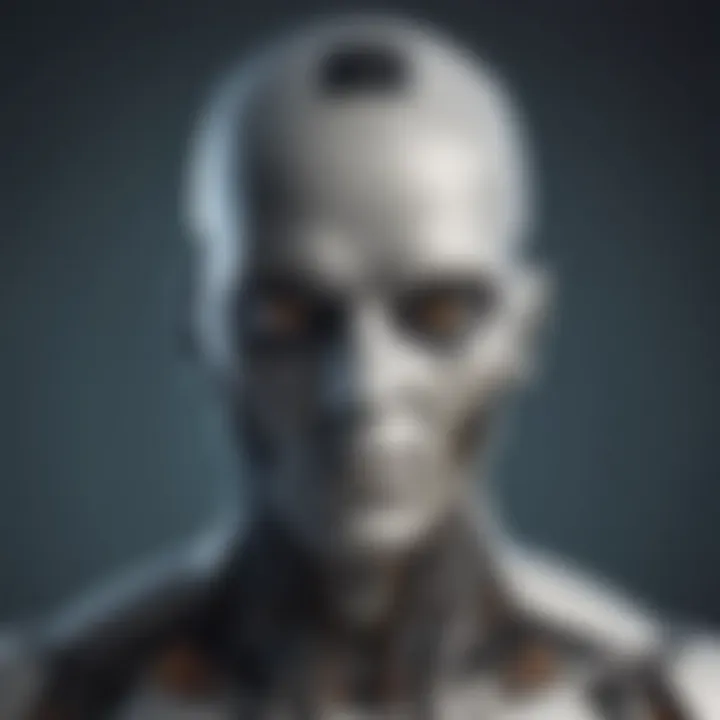
Artificial Intelligence (AI) in networking is at the forefront of technological innovation, driving significant advancements and shaping the future landscape of network optimization, cybersecurity, and data management. The evolution of AI algorithms is crucial in revolutionizing the efficiency and security of networks. Among these advancements, the prospects of Machine Learning (ML) algorithms stand out, offering unparalleled capabilities in addressing complex network challenges. Deep Learning Applications, a prominent subset of ML, play a pivotal role in enhancing network performance through in-depth analysis and pattern recognition. The inherent ability of Deep Learning to uncover hidden insights within vast datasets makes it an indispensable tool in optimizing network operations.
Reinforcement Learning Models, another facet of AI, contribute substantially to network adaptability and automation. By enabling systems to learn from interactions with the network environment, Reinforcement Learning fosters autonomous decision-making and continuous improvement. The iterative learning process of Reinforcement Learning equips networks with the flexibility to adapt to dynamic circumstances and optimize performance, making it a strategic choice for enhancing network efficiency in this evolving technological landscape.
Advancements in Machine Learning Algorithms
Deep Learning Applications
Deep Learning Applications exemplify the pinnacle of AI capabilities in network handling, offering intricate data processing mechanisms and sophisticated analytical tools. Their emphasis on hierarchical feature learning enables networks to extract complex patterns and make data-driven decisions with precision. The deep neural networks underlying Deep Learning Applications allow for multi-level abstractions, empowering networks to streamline processes and enhance overall performance. The adaptability and scalability of Deep Learning Applications make them a valuable asset in optimizing network functionalities, although their resource-intensive nature may pose challenges in certain contexts.
Reinforcement Learning Models
Reinforcement Learning Models introduce a dynamic dimension to network optimization, harnessing adaptive learning techniques to improve network behavior and outcomes. By utilizing a trial-and-error approach, Reinforcement Learning Models fine-tune network strategies according to environmental feedback, continuously refining decision-making processes for optimal results. The reinforcement loop inherent in these models drives iterative improvements, ensuring that networks evolve and adapt in real-time to varying conditions. While offering invaluable benefits in network automation and optimization, the complexity of training and fine-tuning Reinforcement Learning Models demands substantial computational resources and expertise to leverage effectively.
Integration of AI with IoT and Edge Computing
Smart Connectivity Solutions
The integration of AI with Internet of Things (IoT) and Edge Computing heralds a new era of intelligent connectivity solutions, catering to the growing demands of interconnected devices and systems. Smart Connectivity Solutions leverage AI algorithms to facilitate seamless communication and data exchange between IoT devices, optimizing connectivity pathways and enhancing network efficiency. By intelligently managing data traffic and prioritizing critical information, these solutions pave the way for enhanced IoT functionality and streamlined network operations. However, the extensive connectivity and data processing capabilities of Smart Connectivity Solutions may introduce complexities regarding data security and privacy, necessitating robust safeguards and encryption protocols.
Edge Intelligence Implementation
Edge Intelligence Implementation represents a paradigm shift in network architecture, distributing computational capabilities closer to data sources for real-time processing and decision-making. By decentralizing AI algorithms to the network's edge, Edge Intelligence minimizes latency and enhances responsiveness, empowering networks to deliver rapid data insights and actionable intelligence. The agility and responsiveness of Edge Intelligence Implementation elevate network performance and reliability, especially in time-sensitive applications and dynamic environments. Nevertheless, the distributed nature of edge computing poses challenges in maintaining consistent data integrity and synchronization across interconnected devices, necessitating robust synchronization protocols and fault-tolerant mechanisms.
Ethical Considerations in AI Network Development
Data Privacy Regulations
The ethical implications of AI network development highlight the critical importance of implementing stringent data privacy regulations to safeguard sensitive information and uphold user rights. Data Privacy Regulations play a pivotal role in governing data collection, storage, and utilization within networks, ensuring transparent practices and accountability. By adhering to privacy regulations and frameworks, organizations can cultivate trust and compliance among users while mitigating the risks of data breaches and security vulnerabilities. However, the enforcement and implementation of stringent data privacy regulations may introduce compliance challenges and operational complexities for network stakeholders, necessitating a balance between data protection and operational efficiencies.
Bias Mitigation Strategies
Bias Mitigation Strategies address the ethical considerations surrounding algorithmic biases and discriminatory practices within AI network development. By proactively identifying and mitigating biases in AI algorithms, organizations can foster fairness, equity, and transparency in network operations. These strategies encompass algorithmic audits, bias detection mechanisms, and diverse dataset curation techniques to minimize biases and promote inclusivity. Despite their crucial role in promoting ethical AI development, the implementation of Bias Mitigation Strategies requires continuous monitoring, evaluation, and adjustment to adapt to evolving datasets and contextual dynamics, underscoring the ongoing commitment to ethical AI practices and principles.