Uncovering Insights: Navigating the Online Landscape for Unstructured Data Discovery
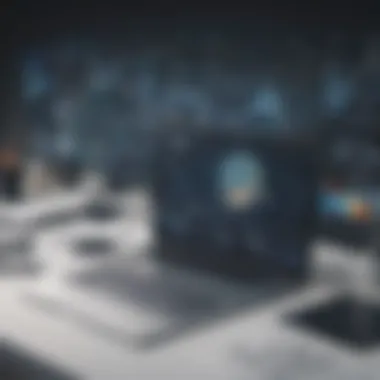
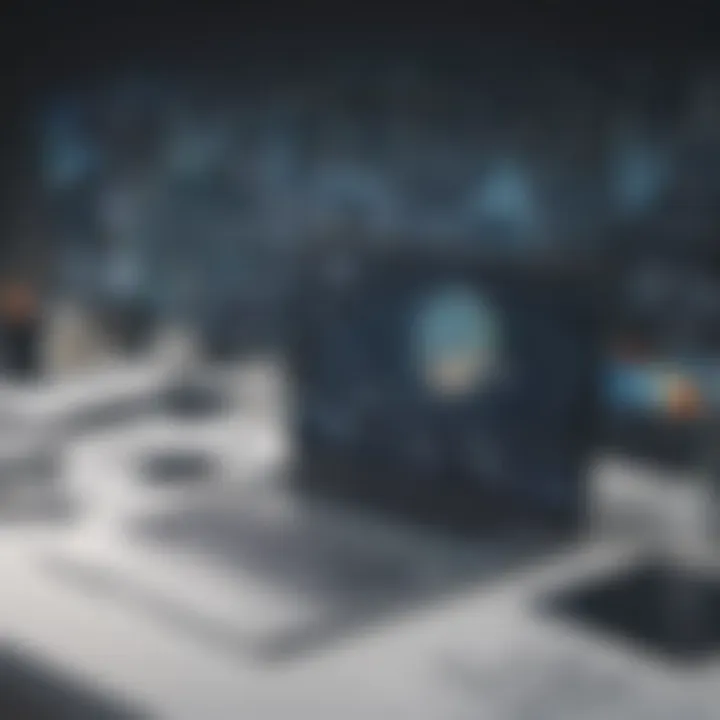
Overview of Cyber Security Threats
Cyber security threats are a prevalent concern in today's digital landscape, posing risks to individuals' online security and privacy. Malware, phishing, and ransomware are among the common types of cyber threats individuals may encounter. These malicious entities target sensitive information, leading to potential data breaches and financial losses. Additionally, statistics on cyber attacks reveal a disturbing increase in both frequency and sophistication. Real-life examples of security breaches serve as cautionary tales, emphasizing the importance of robust security measures to navigate the digital realm safely.
Best Practices for Online Security
Implementing best practices for online security is crucial in fortifying one's digital defenses. Strong password creation and management techniques, such as using complex and unique passwords for each account, are fundamental steps in preventing unauthorized access. Regular software updates and patches play a vital role in addressing known vulnerabilities, reducing the risk of exploitation by cyber threats. Furthermore, embracing two-factor authentication bolsters security by adding an extra layer of verification, enhancing account protection against unauthorized entry.
Reviews of Security Tools
When evaluating security tools, considerations must be made based on their effectiveness in safeguarding against potential threats. Antivirus software is a key component in detecting and eliminating malware, offering essential protection for devices. Comparing firewall protection mechanisms aids in determining the most robust defense against external intrusions. Additionally, the assessment of password managers is vital for securely storing and managing credentials, promoting convenient yet secure access to various accounts.
Tips for Ensuring Online Privacy
Protecting online privacy is paramount in preserving confidentiality and preventing data misuse. Using virtual private networks (VPNs) enables secure browsing by encrypting internet traffic, shielding users from prying eyes. Adjusting privacy settings on social media platforms allows individuals to control the visibility of their personal information, limiting exposure to potential threats. During online transactions, safeguarding personal data through secure payment gateways and encrypted connections minimizes the risk of sensitive information falling into the wrong hands.
Educational Resources and Guides
Educational resources and guides provide valuable insights into enhancing online security practices. Articles detailing the setup of encryption tools offer step-by-step instructions on fortifying data protection. Guides on identifying and avoiding phishing emails equip individuals with the knowledge to recognize and report suspicious activities, reducing the likelihood of falling victim to cyber fraud. Cheat sheets compiled with quick tips on reinforcing online security serve as handy references for maintaining a vigilant stance against evolving cyber threats.
Introduction to Unstructured Data Discovery
Unstructured Data Discovery serves as a critical component in navigating the digital landscape safely, delving into the vast realm of data complexities that traditional methods struggle to unearth. By shedding light on data in its rawest form, this section introduces readers to the foundational understanding needed to traverse the intricacies of unstructured data. Through a comprehensive examination of unstructured data discovery, individuals gain invaluable insights into safeguarding their online presence effectively.
Defining Unstructured Data
Characteristics of Unstructured Data
When dissecting the Characteristics of Unstructured Data, one encounters a landscape rich in diversity and complexity. The fundamental aspect lies in the lack of predefined structure, where data does not fit neatly into traditional databases. This aspect challenges conventional data processing methods but offers unparalleled flexibility in accommodating a wide array of data types and formats. Such dynamism allows for a comprehensive exploration of data nuances, fostering a deeper understanding of intricate data relationships within a digital space.
Unstructured Data's hallmark versatility presents both advantages and disadvantages in the realm of data discovery. While traditional structured data may offer easy categorization, unstructured data's fluid nature enables a more holistic representation of real-world scenarios. However, this flexibility can complicate data management and analysis, requiring specialized tools and techniques to derive meaningful insights effectively.
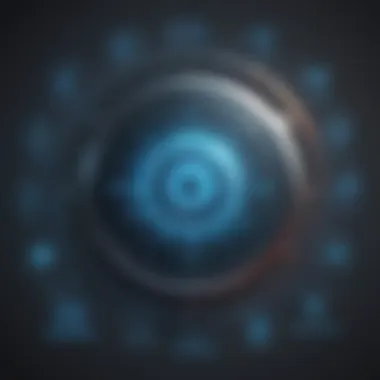
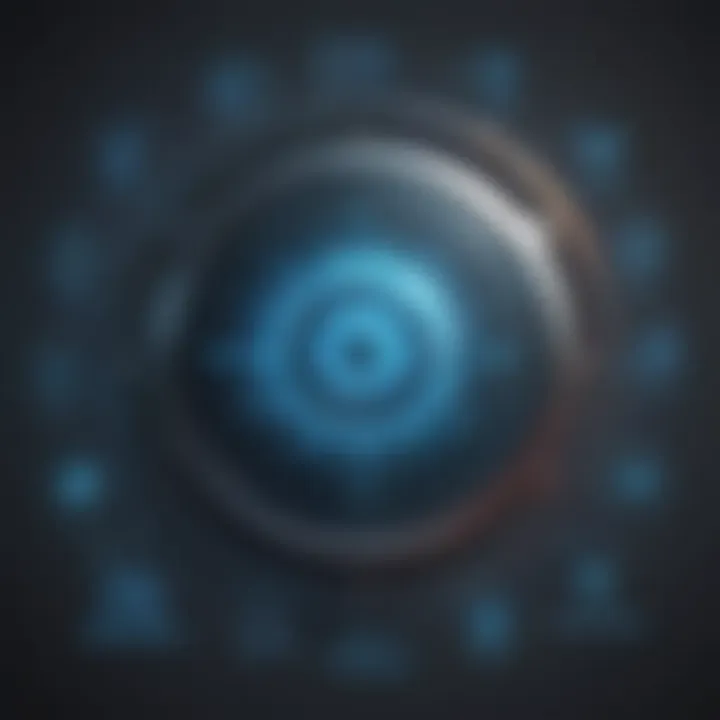
Examples of Unstructured Data
Examples of Unstructured Data exemplify the varied forms and sources from which unstructured data emanates. It includes text data from social media posts, emails, audio files, video content, and more. The presence of unstructured data underscores the vast landscape that organizations must navigate to gain a comprehensive understanding of their digital footprint.
Delving into diverse data forms not only expands the breadth of insights available but also challenges traditional data processing paradigms. The richness of Examples of Unstructured Data lies in the potential to uncover hidden patterns and correlations that structured data alone might overlook. Embracing these varied data sources empowers individuals to extract actionable intelligence, enabling informed decision-making within the digital domain.
Significance of Unstructured Data Discovery
Impact on Online Security
Impact on Online Security underscores the pivotal role unstructured data plays in fortifying digital defenses. By leveraging insights obtained from unstructured data, organizations can proactively identify potential security threats and vulnerabilities. The malleability of unstructured data allows for comprehensive risk assessment, enhancing cyber resilience and safeguarding sensitive information from malicious actors.
Revealing the nuanced interplay between data patterns and security risks, Impact on Online Security elevates online threat detection to a proactive realm. Through detailed analysis and anomaly detection, organizations can preempt cyber attacks and ensure data integrity in an increasingly digitized landscape.
Relevance to Privacy Protection
The Relevance to Privacy Protection underscores the critical link between unstructured data exploration and safeguarding personal information. By unraveling the intricacies of unstructured data, individuals can gain a comprehensive view of the digital trail they leave behind. This heightened visibility enables proactive privacy measures and empowers users to control the dissemination of their personal data.
Empowering individuals to make informed decisions about data sharing and privacy settings, Relevance to Privacy Protection fosters a culture of data stewardship and digital accountability. By appreciating the value of unstructured data in fortifying privacy defenses, individuals can navigate the digital landscape with confidence, knowing that their privacy remains safeguarded amidst evolving cyber threats.
Challenges in Unstructured Data Discovery
Unraveling the complexities of unstructured data discovery within the digital landscape is paramount in ensuring online security and privacy. By delving into the challenges presented in this sphere, we equip ourselves with the necessary knowledge to navigate this intricate terrain effectively.
Volume and Variety of Data
The sheer volume and diverse nature of data in the unstructured realm pose significant hurdles in the quest for data discovery. Addressing the management of large data sets is a critical aspect that requires meticulous attention. Managing large data sets involves handling vast amounts of information efficiently to derive meaningful insights. This process plays a pivotal role in the overall unstructured data discovery, influencing decision-making and strategic planning significantly.
Dealing with diverse data formats is another crucial challenge that demands consideration. The multitude of data formats adds layers of complexity to the analysis and interpretation of unstructured data. Understanding how to navigate through these diverse formats is essential for extracting valuable insights and patterns effectively. While this aspect enhances the depth of exploration, it also introduces intricacies that necessitate a nuanced approach to data processing.
Lack of Structure and Organization
Within the realm of unstructured data, the lack of predefined structures and organization presents formidable challenges to data discovery efforts. Discussing issues with data classification sheds light on the obstacles encountered in categorizing and organizing unstructured data. The ambiguity surrounding data classification complicates the identification and extraction of pertinent information, impacting the overall effectiveness of data discovery processes.
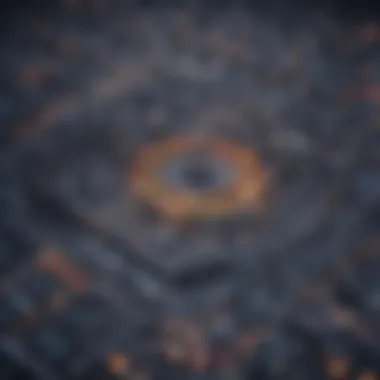
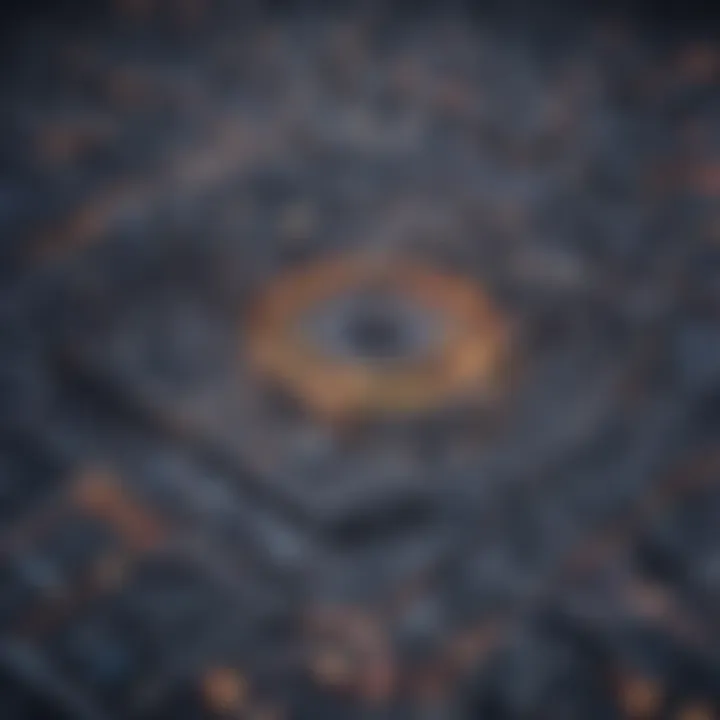
Concurrently, the difficulty in data retrieval stands as a significant barrier in harnessing the full potential of unstructured data. The challenges in retrieving relevant data hamper the efficiency of analysis and impede decision-making processes. Addressing these retrieval obstacles is crucial in streamlining data discovery procedures and unlocking valuable insights hidden within unstructured data repositories.
Techniques for Unstructured Data Discovery
Data Mining and Text Analytics
Extracting Insights from Textual Data
The aspect of Extracting Insights from Textual Data plays a pivotal role in unraveling valuable information hidden within unstructured data. By harnessing data mining and text analytics tools, individuals can derive significant insights from textual content, enabling a deeper understanding of online security threats and privacy concerns. The key characteristic of Extracting Insights from Textual Data lies in its ability to uncover patterns and anomalies within large sets of unstructured information. This method is a favored choice in the quest for online security as it assists in identifying potentially malicious content or unauthorized access attempts. However, a potential drawback of this approach may involve the complexity of analyzing diverse textual formats, posing challenges in efficient data processing.
Identifying Patterns and Trends
The identification of Patterns and Trends within unstructured data is paramount in recognizing recurring themes or behaviors that may impact online security measures. By actively seeking and interpreting patterns, individuals can stay ahead of emerging threats and proactively address vulnerabilities in their digital systems. Identifying Patterns and Trends showcases a key characteristic of foreseeing potential risks or irregularities before they escalate into significant security breaches. This technique proves advantageous in pinpointing unusual activities or trends that deviate from established norms, enabling timely intervention to mitigate risks. Nonetheless, the process of identifying patterns and trends may require sophisticated algorithms and continuous refinement to adapt to evolving cybersecurity landscapes.
Natural Language Processing (NLP)
Understanding Human Language Data
The engagement with Understanding Human Language Data is pivotal in enhancing data interpretation capabilities within the domain of unstructured data discovery. Through natural language processing techniques, individuals can decipher complex text structures and extract meaningful insights from linguistically diverse sources. The main characteristic of Understanding Human Language Data lies in its ability to decode and interpret human-generated content, offering a comprehensive view of online interactions and communications. This feature proves valuable in identifying linguistic patterns indicative of potential security threats or privacy breaches. However, the reliance on natural language processing may pose challenges in accurately interpreting context-dependent language nuances, potentially leading to misinterpretations or false positives.
Enhancing Data Interpretation
Enhancing Data Interpretation through natural language processing methodologies contributes significantly to refining data analysis and decision-making processes. By harnessing NLP tools, individuals can enhance their understanding of nuanced language structures and sentiments present in unstructured data sets, enabling more precise data interpretations. The distinctive feature of Enhancing Data Interpretation lies in its capacity to refine data models and uncover subtle correlations within textual information, offering a deeper insight into online security vulnerabilities. This approach proves beneficial in fine-tuning threat detection mechanisms and strengthening privacy protection measures. Yet, challenges may arise in balancing computational efficiency with the nuanced complexities of human language, requiring continuous optimization and training of NLP models to ensure accurate data interpretation.
Tools for Unstructured Data Discovery
In the vast landscape of unstructured data discovery, the utilization of tools holds immense importance. Tools for unstructured data discovery play a pivotal role in unlocking valuable insights from data sets that lack a defined structure. These tools serve as the bridge between raw data and meaningful interpretations, offering functionalities to extract, analyze, and visualize data efficiently. Through the integration of various tools, individuals can streamline their data discovery process, leading to informed decision-making and strategic insights that can bolster online security and privacy efforts.
Data Visualization Software
Visualizing Complex Data Sets
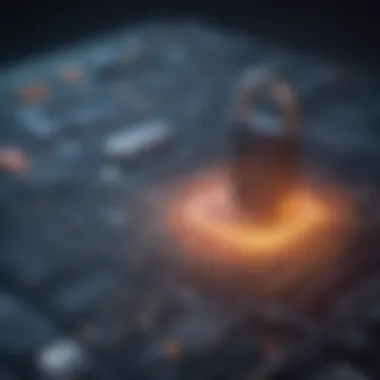
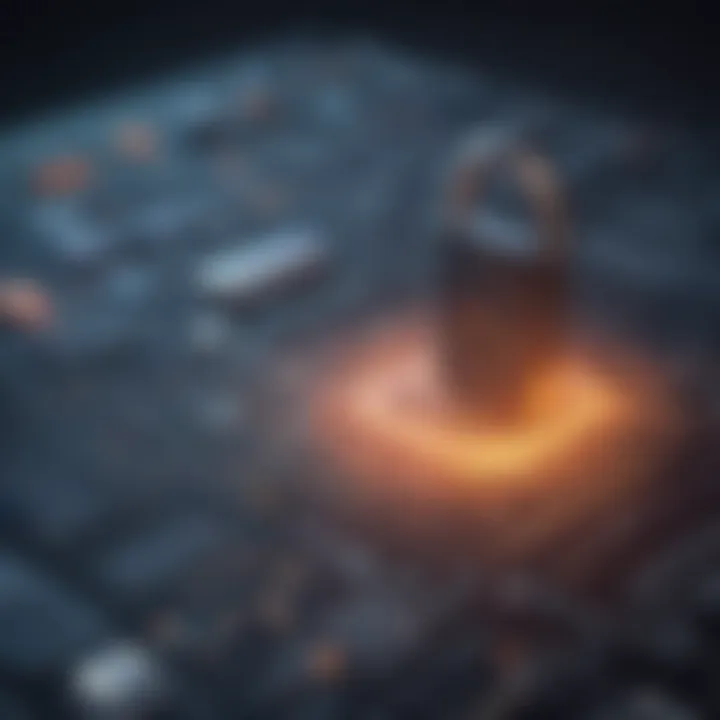
Data visualization software plays a crucial role in the unstructured data discovery realm by enabling users to represent intricate data sets in a visually comprehensible format. The key characteristic of visualizing complex data sets lies in its ability to transform large volumes of unstructured data into interactive charts, graphs, and dashboards, facilitating a deeper understanding of underlying patterns and trends. This visual representation enhances data exploration processes, allowing individuals to uncover hidden correlations and anomalies efficiently. The unique feature of visualizing complex data sets is its capacity to simplify complex data structures, making intricate relationships more accessible to users. While offering a visually appealing and intuitive data representation, visualizing complex data sets may require a learning curve for users unfamiliar with interpreting visualized data.
Facilitating Data Exploration
Facilitating data exploration is a fundamental aspect of data visualization software that contributes significantly to the overall goal of unstructured data discovery. This feature empowers users to interact with data dynamically, enabling them to explore different dimensions of the data set and gain comprehensive insights. The key characteristic of facilitating data exploration is its user-friendly interface, which allows individuals to interact with data points, apply filters, and delve deeper into specific data segments seamlessly. By providing a hands-on exploration experience, this aspect fosters a deeper understanding of data patterns and relationships, promoting faster decision-making and hypothesis testing. The unique feature of facilitating data exploration is its adaptability to various user preferences and data requirements, offering customization options to tailor the exploration process according to specific analytical needs. While facilitating data exploration enhances user engagement and interactivity, it may require adequate training to leverage its full potential effectively.
Machine Learning Algorithms
Automating Data Analysis
Machine learning algorithms play a critical role in automating data analysis tasks within the realm of unstructured data discovery. Automating data analysis simplifies the process of extracting meaningful insights from vast data sets by implementing algorithmic models that can identify patterns and trends autonomously. The key characteristic of automating data analysis is its efficiency in processing large volumes of unstructured data rapidly, saving time and resources for data analysts. By leveraging machine learning algorithms for automated analysis, organizations can efficiently derive actionable insights from complex data sets, leading to informed decision-making and strategy formulation. The unique feature of automating data analysis is its adaptability to evolving data patterns, constantly updating models to improve accuracy and predictive capabilities. While offering valuable time-saving benefits, automating data analysis requires continuous monitoring and validation to ensure the accuracy and relevance of generated insights.
Predictive Modeling
Predictive modeling represents a pivotal aspect of machine learning algorithms in unstructured data discovery, aiming to forecast future trends and outcomes based on historical data patterns. The key characteristic of predictive modeling lies in its predictive accuracy, providing organizations with valuable insights into potential scenarios and risks. By leveraging predictive modeling techniques, businesses can anticipate market trends, customer behavior, and potential risks, thereby optimizing decision-making processes and resource allocation. The unique feature of predictive modeling is its ability to generate probabilistic forecasts and scenario analyses, enabling organizations to mitigate risks and capitalize on emerging opportunities. While offering valuable predictive insights, predictive modeling requires continuous refinement and validation to align with changing business environments and evolving data sources.
Best Practices for Secure Unstructured Data Discovery
In this section, we delve into the critical aspect of implementing best practices for secure unstructured data discovery. Addressing the complexities of safeguarding sensitive information in the digital landscape, it is imperative to employ robust strategies to mitigate risks effectively. By following established best practices, individuals can significantly enhance their online security posture and ensure the privacy of their personal data. Emphasizing key elements such as regulatory compliance, encryption measures, and proactive monitoring, this segment outlines essential steps to fortify data protection.
Ensuring Data Privacy Compliance
Adhering to Data Protection Regulations
Adhering to data protection regulations is a cornerstone of data privacy compliance. By adhering to stringent regulatory frameworks such as GDPR, CCPA, or HIPAA, organizations demonstrate their commitment to upholding the rights of data subjects and maintaining data integrity. The key characteristic of adhering to data protection regulations lies in its comprehensive approach to data governance, encompassing data collection, processing, and storage practices. Compliance with such regulations is a beneficial choice for this article as it underscores the importance of legal adherence in safeguarding unstructured data. The unique feature of adhering to data protection regulations is its holistic nature, covering various aspects of data handling to ensure transparency and accountability. While advantageous for data security and privacy, adherence to regulations may pose challenges in terms of operational agility and resource allocation.
Implementing Encryption Measures
Implementation of encryption measures is pivotal in securing sensitive data against unauthorized access. By encrypting data at rest and in transit, organizations can prevent data breaches and protect confidential information from malicious entities. The key characteristic of implementing encryption measures is its role in transforming plaintext data into ciphertext, rendering it unintelligible to unauthorized users. This approach is a popular choice for enhancing data security as encryption serves as a robust defense mechanism against cyber threats. The unique feature of implementing encryption measures is its versatility in safeguarding data across different platforms and communication channels. While advantageous for data protection, encryption measures may introduce complexity in data handling and require specialized expertise for implementation.
Regular Data Audits and Monitoring
Monitoring Data Access
Monitoring data access is vital for detecting and preventing unauthorized activities that may compromise data security. By closely monitoring user interactions and access privileges, organizations can identify anomalous behavior and mitigate risks promptly. The key characteristic of monitoring data access is its real-time visibility into data usage patterns, enabling swift responses to potential security incidents. This practice is a beneficial choice for this article as it underscores the importance of proactive security measures in safeguarding unstructured data. The unique feature of monitoring data access is its ability to generate comprehensive audit trails and activity logs, facilitating thorough incident investigations and compliance audits. While advantageous for threat mitigation, monitoring data access may require sophisticated monitoring tools and continuous oversight to maintain effectiveness.
Identifying Anomalies
Identifying anomalies involves detecting deviations from expected data patterns or behaviors within a system. By leveraging anomaly detection techniques such as machine learning algorithms or statistical analysis, organizations can pinpoint irregularities that may signify security breaches or data misuse. The key characteristic of identifying anomalies is its proactive stance towards data security, enabling early detection of potential threats before they escalate. This approach is a popular choice for enhancing anomaly detection capabilities and strengthening overall data protection strategies. The unique feature of identifying anomalies is its ability to adapt to evolving threat landscapes and diverse data sources, enhancing situational awareness and response readiness. While advantageous for threat detection, identifying anomalies may lead to false positives or require fine-tuning to reduce operational overhead.
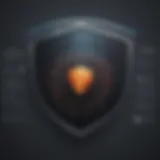
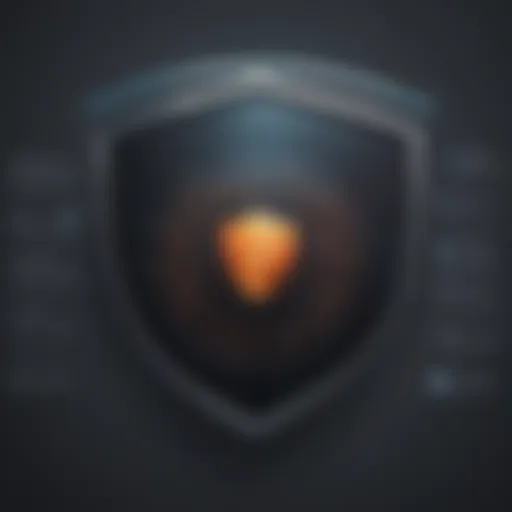